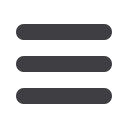
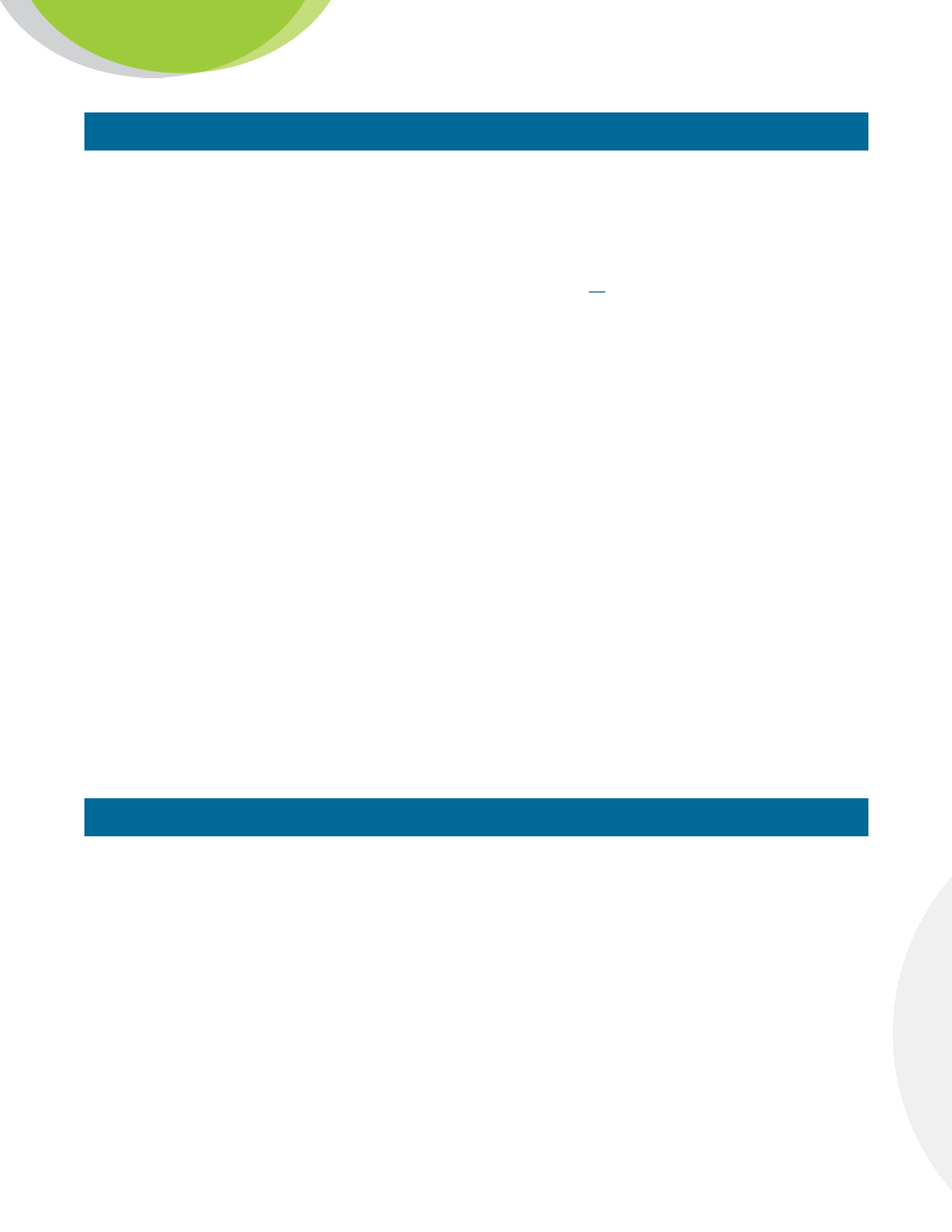
W H I T E P A P E R
www.persistent.com
© 2017 Persistent Systems Ltd. All rights reserved.
4
2. Why analytics industry is slow in moving to the cloud
Deploying applications in the cloud have become normal in the enterprises, especially for CRM and customer
facing applications. However, analytics applications, which require data from various data sources, have not
been deployed in the cloud frequently. We believe this obeys to several reasons:
1. The first and foremost concern is
security
and
privacy.
Enterprises are worried about their customer’s
data privacy and related regulated laws around it. This has been identified as the top obstacle to
implementing analytics in the cloud, according to market survey
[1] .Before moving to cloud they would
like cloud service providers to provide assurance on data security and privacy.
2. While there were guidelines and
compliance regulations
governing data, issued by standards bodies
and governments, it was not possible to have data reside outside your premise. However, regulatory
agencies and standards bodies have recognized the value and popularity of cloud services. New
guidelines and compliance updates are spelling out safe use of the cloud. Adoption of cloud analytics
will certainly increase as these guidelines get implemented at cloud platform providers, and as these
providers offer co-located data centers in the country.
3. Due to queries running over large volume of data,
performance
is critical in some business analytics
scenarios. Cloud deployments can only add to the latency.
4. Even after accepting all above points, some enterprises may raise eyebrows on
data movement
, as it
is really a big challenge to move tens and hundreds of terabytes of data from the on premise to cloud
environments. Cloud providers have come up with offline as well as online data integration tools to help
solve this issue. But the real challenge is to make data movement to and from the cloud a seamless part
of the enterprise data flow. For cloud analytics, enterprises must think about two-way data movement
using streaming data pipelines.
5. There is also a belief that
cloud products feature set
does not match the features of their respective on
premise products. While this may be true for few products, most cloud products currently provide better
product features and support than on premise version.
6. Enterprises call cloud implementations as
black boxes
as they lose visibility and control over it. At times,
they find it hard to tune or configure parameters which they could easily setup when everything is in their
control.
7. Last but not the least, don’t forget the
investments
already made by enterprises in on premise
deployments. There must be real ROI before them moving to cloud. Usually these should be planned
along with major hardware/upgrade cycles.
3. Factors to consider for Cloud Analytics
This section lists a variety of factors that must be considered when moving an analytics implementation to the
cloud or deploying a new implementation. Factors are grouped in four categories:
(i) Requirements on the
data
used to derive analytics insights (beyond big data’s popular “three V’s”, the
types of data and the needs for integration, and quality);
(ii) Requirements on the
queries
needed to derive such insights (whether they are known or not, their type,
analytic workload, response times and user scales issuing these queries);
(iii)
Non-functional
requirements such as security, compliance, performance and scalability, management of
resources in the cloud, and consistency model under update failure (if the analytics solution is embedded
in or deeply related to a read-write application); and
(iv)
Business
requirements such as internal technical and end-user skills, cost, and pricing model.